Say you’re about to launch a music artist’s new album. Is there any way to predict how well it will sell, or how soon the album’s single will hit the charts?
Companies in both the music and entertainment industries often rely upon human judgment—that of marketers, rather than statistical analysis—to forecast demand for these products. How effective is that, really? And what might make it more effective?
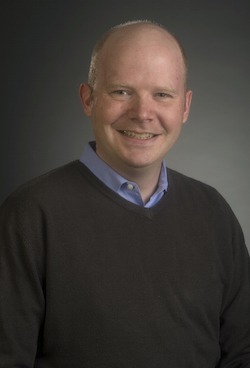
I worked with Matthias Seifert of IE University, Allègre L. Hadida of the University of Cambridge, and Andreas B. Eisingerich of Imperial College to study the role of human judgment in demand forecasting within the music industry.
In the paper, we refer to songs and films as “fashion products” because of the fickleness of audiences and the difficulty of predicting a song or film’s success.
Previous research has shown that people are much better than statistical models at recognizing and understanding complex, contextual interactions, which suggests that people are most effective at predicting the popularity of a song or a film. So how can companies make the best use of these forecasters’ judgment?
Our team interviewed 23 senior managers at four major record companies to identify the variables they used to predict a song’s success: the marketing budget for each single, which other singers were releasing albums at the same time as a particular artist, and whether the artist being analyzed was an established singer or new to the industry.
First the bad news–it’s very hard for human forecasters to beat a statistical model that uses this data. This does not mean, however, that human forecasters do not provide value. While the statistical methods may struggle with identifying non-linear relationships between these variables, human judgment may be better suited to understand how one variable only plays a role in the presence of another. In particular, we found that contextual information, such as the amount of promotion a song gets, whether the song has an accompanying video, what early reviewers write, and how customers seem to be reacting to the product, improves forecasters’ ability to understand such non-linearities in the data.
On the other hand, historical data, such as previous sales of an artist’s work, introduces biases that negatively influence the ability of the forecaster to spot non-linearities. Historical data works well when entered into a statistical model, but it distracts human forecasters from their main task. This is a tough sell, because most forecasters want all the information they can get. After all, we’re trained to think more information is better, to help us make better-informed decisions. Our research reveals that too much data, particularly historical data, can lead people tasked with predicting the success of a film or song astray.
For the highest rate for forecasting success, music and entertainment companies should provide people with contextual data and feed historical data into statistical models, which can more effectively process that type of information. The result will be two forecasts—a statistical forecast and a judgment forecast—and then organizations can find ways to reconcile those two forecasts. The simplest way to do it is to average them, which is often not a bad thing, but there are also ways to find optimal weights between them.
In short, when it comes to demand forecasting data, it turns out that more is not necessarily better.
For more on this topic, see our paper “Effective Judgmental Forecasting in the Context of Fashion Products” in The Journal of Operations Management.
Enno Siemsen is Fetzer Family Chair in Business Fund; Procter & Gamble Bascom Professorship; executive director of the Erdman Center for Operations & Technology Management; professor of operations & information management at the Wisconsin School of Business.
Tags: