Suppose you are the manager of a local zoo. Every year you purchase standard building insurance to protect your assets. Your insurance company also offers “zoo animal coverage” at an additional cost.
Many insurance policies offer these additional coverage options, known as “endorsements,” or “riders,” which include alternative deductibles and coverage limits.
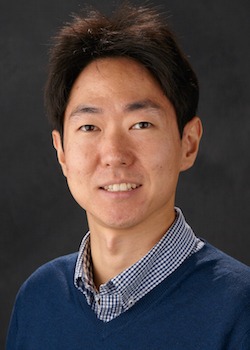
How do insurance companies determine the price of the additional premium? Because endorsements are optional and typically inexpensive compared to primary coverage, data about them can be sparse. When data is limited in this way, standard methods may suggest unreasonable rates, and premiums might end up being too high. Adjusters, therefore, tend to set endorsement prices on a one-off basis.
Professor Jed Frees, my Ph.D. advisor, and I wanted to improve the way endorsements are rated by providing interpretable, reasonable rates in a data-driven way. Our aim was to create a state-of-the-art statistical system to improve insurance pricing.
What impact would such a system have on the insurance industry? For actuarial analysts, it would provide a practical way of solving a real-world problem. We wanted to find a way to evaluate endorsements more efficiently, saving valuable work time.
We evaluated the Wisconsin Local Government Property Insurance Fund (LGPIF) to come up with a process for determining intuitively appealing rates in a political context, based on generalized linear modeling (GLM) techniques.
The LGPIF provides property insurance for local government entities, like cities and school districts, and acts as a stand-alone insurance company, charging premiums to each policyholder and paying claims when appropriate. There is little in the literature on government property applications for general insurance, so we wanted to contribute to that body of research.
We began by analyzing the property fund data and researching the best statistical method to apply to our data set. Once we identified an effective approach, we built and tested a model, using statistical computing.
For a statistical audience, our research is an interesting application of a method in a specified context: To accommodate potentially conflicting goals of data complexity and algorithmic transparency, we utilized shrinkage techniques to moderate the effects of endorsements with penalized likelihoods.
This approach can be understood as a special case of constrained estimation, where the coefficients are restricted to be within a small neighborhood of the target. Our method avoids ad-hoc adjustments and uses a data-driven approach to the endorsements problem.
The results were consistent with our prediction. We found that using shrinkage methods lost little predictive ability, and they gave much more intuitively appealing relativities. In a politically sensitive environment like government insurance, it is helpful to have relativities that can be calibrated in a disciplined manner and are consistent with sound economic, risk management, and actuarial practice.
Compared to the traditional method of simply including endorsements after the primary analysis has been done, our approach allows us to use the data to suggest ways of introducing relativities for endorsements. Also, because we use GLM techniques, our approach is naturally multivariate, and the introduction of endorsements accounts for the presence of other rating variables. In addition, the shrinkage methods we used are flexible enough to be used in other situations in which an analyst wants to moderate the effect of unreliable data.
Our method constrains each endorsement premium within an acceptable range, without sacrificing the predictive ability of the overall rating engine. This would make our hypothetical zoo manager happier, and the insurance company would be happy, too. So in some ways, our study is an attempt to provide a win-win insurance solution for ordinary people. In fact, our method made so much sense in practice that the LGPIF ratemaking team is currently using it.
We have included a detailed case study in our paper, so that other analysts may replicate parts of our approach. For more on this topic, see our paper “Rating Endorsements using Generalized Linear Models,” forthcoming in Variance.
Statistical methods are evolving, and regularization methods are improving. We’re interested in finding out if it’s possible to improve our practices using more advanced models and methods. New regularization methods and claims triaging methods are two interesting topics for future research. How would these advanced methods affect the risk measure of an insurance company? These compelling questions remain to be explored.
Tags: